❓ #DidYouKnow that when overwhelmed by information, you can use #statistics to help you decide what to believe in?
📉 No? Well, then check out #Bayesian statistics. Unless you have studied mathematics, you’ve probably never heard of it before. It is the evil twin of #Frequentist statistics, which you most likely encountered and even feared at school.
📊 Bayes’s theorem is probably the most influential idea in history. It can tell you, for example, what beliefs you should hold, which you should change, and why arguing with a conspiracy theorist will only enforce their beliefs.
🧠 Opposed to the frequentist approach, Bayesian statistics take into account your prior beliefs when comparing multiple hypotheses against each other. And while Frequentists require a lot of data, Bayesians are happy with less when provided with an expert opinion.
🗝️ The key concept of Bayesian inference is to look at all available data and consider your view of the world P(D I H1) and compare it to all other possible views P(D I Hn) before multiplying it by a so-called prior probability ratio. You see the equation of this in the picture.
The prior describes how strongly you or an expert believe in your world 🌎 or how willing you are to change your mind. Here, the value of the prior can take any value you like.
📈 Once you have obtained your posterior probability P(H I D), you can normalise your data by dividing each posterior by the sum of all posterior values and plot it against P(D I Hn). You will immediately see how likely your view of the world is compared to other views and whether you should consider changing it.
⬇️ Of course, as in life, there is also no such thing as a free lunch in statistics. Although Bayesian statistics seem great as it requires less data, the approach also has its downsides. For example, deciding how to select or quantify a prior is very hard, which is especially problematic as it significantly influences the result.
🚙 But having said this, Bayesian inference is probably the closest to human decision-making, taking past experiences into account. It is also the reason why autonomous vehicles, as we know them, are possible. There are obviously many more applications of Bayesian statistics in machine learning, and I encourage you to learn more about this very powerful decision-making tool.
📚 So do check out this amazing #book by Will Kurt, Bayesian Statistics the Fun Way, or episode SDS 581 of the Super Data Science Podcast.
Links to the Sources:
Contributing Editor: Women in AI & Robotics#Berlin community member, Dr. Jana Stucke.
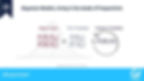